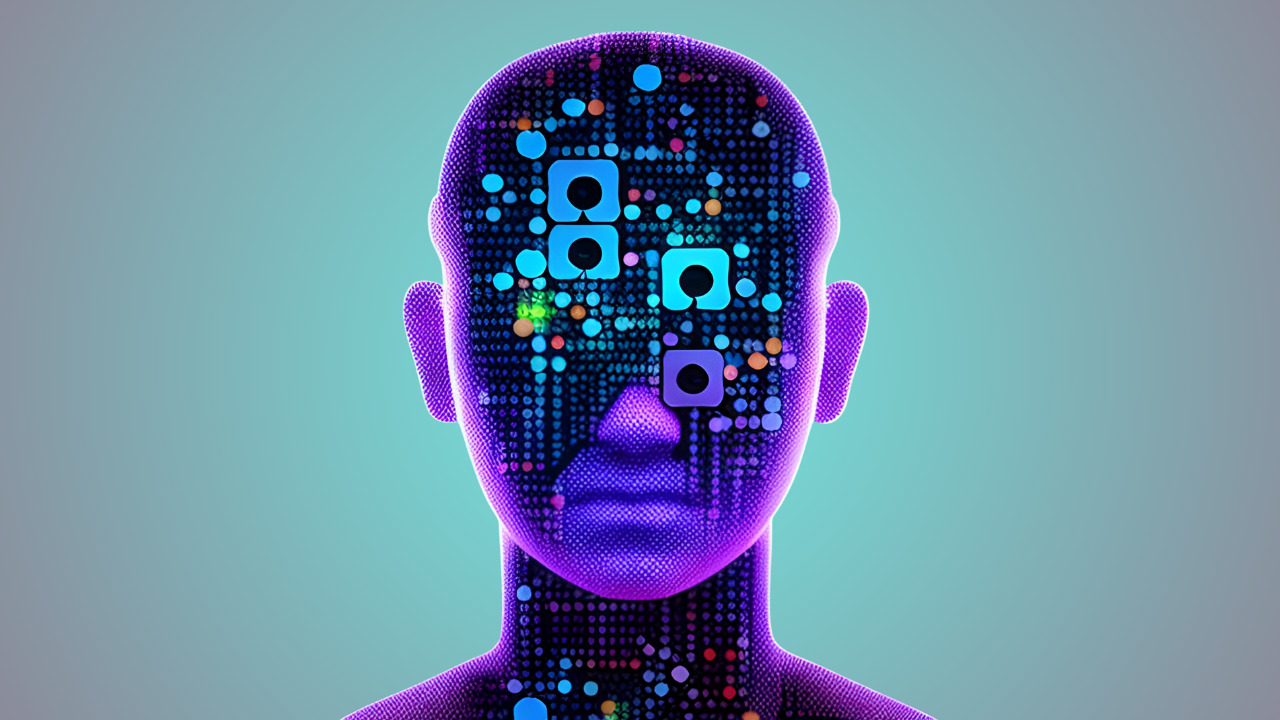
Selçuk DİKİCİ Industrial Engineer, Istanbul University E-mail: selcukdikici@ogr.iu.edu.tr
Abstract
Artificial intelligence (AI) technologies are increasingly integrated into modern software development processes. AI-assisted advanced programming encompasses the effective use of techniques such as machine learning, deep learning, natural language processing, and computer vision in software engineering. This study examines the role of artificial intelligence in software development, AI-based development tools, and its impact on optimization processes. The contributions of AI-assisted programming to automated code generation, debugging, security testing, and decision support systems are analyzed.
Keywords: Artificial Intelligence, Machine Learning, Deep Learning, Natural Language Processing, Computer Vision, Automated Coding, Security
1. Introduction
Artificial intelligence is revolutionizing software engineering by enabling automation in data analysis and decision-making processes. While traditional software development processes are based on rule-based approaches, AI-assisted programming has become more dynamic and adaptive through big data analytics and learning algorithms (Russell & Norvig, 2021).
2. Programming with Machine Learning
Machine learning (ML) optimizes software development by allowing programs to learn from data. The most common ML methods include:
- Supervised learning (Goodfellow et al., 2016)
- Unsupervised learning (Murphy, 2012)
- Reinforcement learning (Sutton & Barto, 2018)
TensorFlow and Scikit-learn are widely used libraries for machine learning in programming (Abadi et al., 2016).
3. Deep Learning-Based Applications
Neural networks, particularly deep learning techniques, are effectively used in big data processing. Prominent deep learning architectures include:
- Convolutional Neural Networks (CNN) (LeCun et al., 1998)
- Recurrent Neural Networks (RNN) (Hochreiter & Schmidhuber, 1997)
- Transformer-based models (GPT, BERT) (Vaswani et al., 2017)
GPU-supported deep learning frameworks, such as PyTorch, enable high-speed complex analysis (Paszke et al., 2019).
4. Software Development with Natural Language Processing (NLP)
NLP is a field of AI used in language-based applications such as intelligent assistants, chatbots, and automatic text summarization. AI-supported programming tools for NLP include:
- OpenAI Codex (automatic code generation) (Chen et al., 2021)
- Google BERT (text processing) (Devlin et al., 2019)
- Spacy (language processing library) (Honnibal & Montani, 2020)
5. AI-Assisted Development Tools
AI-assisted programming tools are designed to enhance developer productivity. These tools include:
- AI-powered debugging (DeepCode, SonarQube) (Pradel et al., 2018)
- Intelligent code completion (GitHub Copilot, TabNine) (Tenny et al., 2021)
- Automated test generation systems (Zeller et al., 2019)
6. Advanced Applications with Computer Vision
AI-based computer vision techniques are used in applications such as object recognition, facial recognition, and scene analysis. OpenCV and YOLO frameworks are fundamental tools for AI-assisted computer vision programming (Redmon et al., 2016).
7. AI in Cybersecurity and Anomaly Detection
AI-based security systems offer significant advantages in detecting anomalies and identifying cyber threats. Machine learning techniques are used to analyze network traffic and automatically classify potential threats (Goodfellow et al., 2016).
8. Conclusion and Future Perspectives
AI-assisted programming has the potential to make software engineering processes more efficient and error-free. In the future, AI systems supported by quantum computing are expected to further enhance software development. Additionally, the widespread adoption of autonomous software development systems may minimize human intervention.
References
- Abadi, M., et al. (2016). TensorFlow: A system for large-scale machine learning. OSDI.
- Chen, M., et al. (2021). Evaluating large language models trained on code. arXiv.
- Devlin, J., et al. (2019). BERT: Pre-training of deep bidirectional transformers for language understanding. NAACL.
- Goodfellow, I., Bengio, Y., Courville, A. (2016). Deep learning. MIT Press.
- Hochreiter, S., Schmidhuber, J. (1997). Long short-term memory. Neural Computation.
- Honnibal, M., Montani, I. (2020). spaCy: Industrial-strength NLP.
- Murphy, K. P. (2012). Machine learning: A probabilistic perspective. MIT Press.
- Paszke, A., et al. (2019). PyTorch: An imperative style, high-performance deep learning library. NeurIPS.
- Pradel, M., et al. (2018). DeepBugs: A learning approach to name-based bug detection. SIGPLAN.
- Redmon, J., et al. (2016). You only look once: Unified, real-time object detection. CVPR.
- Russell, S., Norvig, P. (2021). Artificial intelligence: A modern approach. Pearson.
- Sutton, R. S., Barto, A. G. (2018). Reinforcement learning: An introduction. MIT Press.
- Tenny, R., et al. (2021). AI-assisted code completion: A comparative analysis. ICSE.
- Vaswani, A., et al. (2017). Attention is all you need. NeurIPS.
- Zeller, A., et al. (2019). Automated testing with AI: A survey. ICST